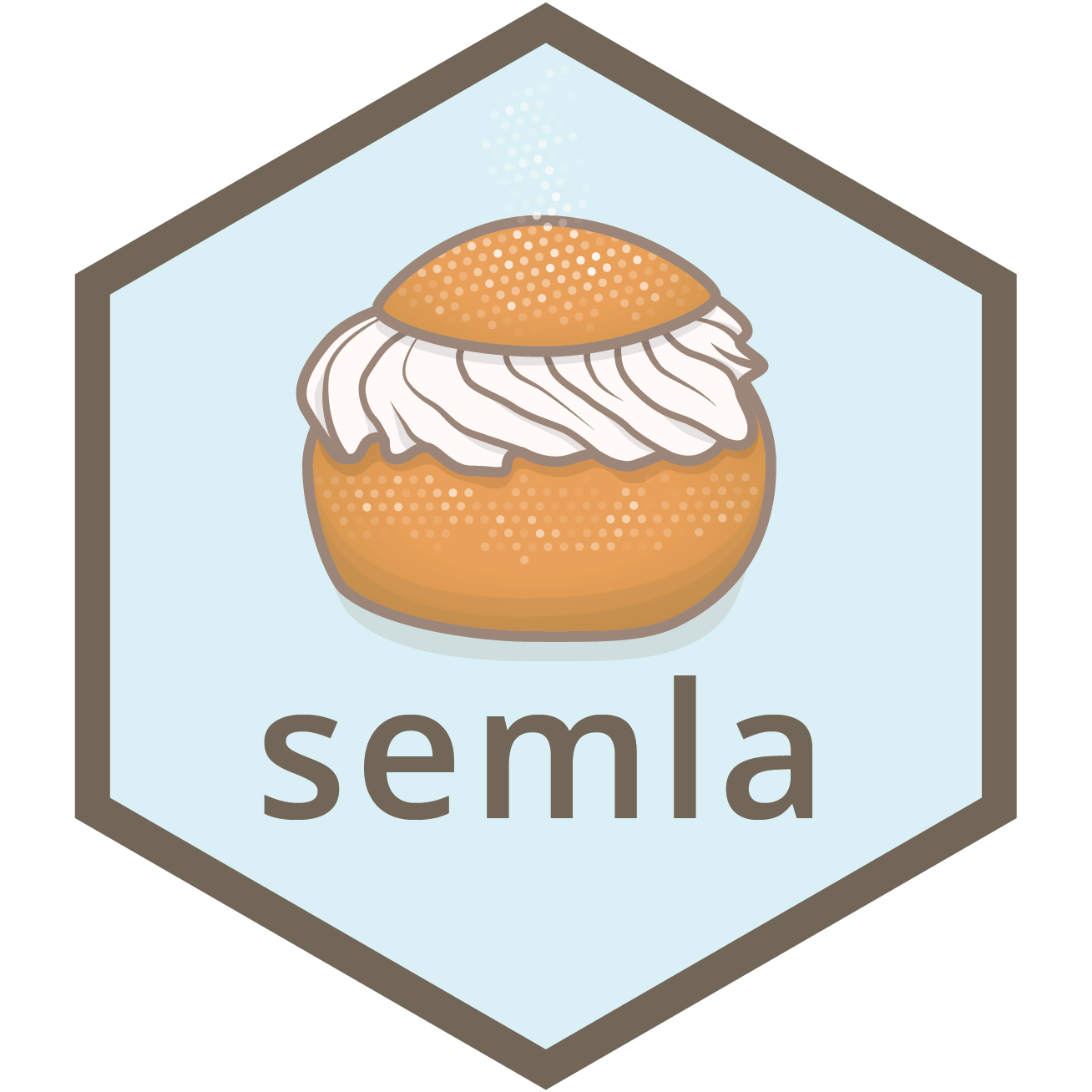
Neighborhood Enrichment Analysis
neighborhood-enrichment.Rd
Performs Neighborhood Enrichment Analysis between spot labels, describing whether spots of two categories lie next to each other spatially more often than expected by chance.
Usage
RunNeighborhoodEnrichmentTest(
object,
column_name,
column_labels = NA,
n_permutations = 200,
nCores = parallel::detectCores() - 1,
seed = 123,
verbose = TRUE
)
Arguments
- object
A Seurat object
- column_name
Column name in metadata corresponding to label ID of the spots.
- column_labels
Optional. Provide vector of label IDs to subset your analysis by. Spots of all other labels will be excluded. Must be more than one. Default is all (NA).
- n_permutations
Integer specifying number of iterations the labels should be randomized [default: 200]. Recommended to increase the number of permutations to >=200 for more robust results. A lower number of permutations will result in high standard deviations and thus more unreliable z-scores.
- nCores
Number of cores [default: parallel::detectCores() - 1]
- seed
A seed for reproducibility [default: 123]
- verbose
Print messages [default: TRUE]
Details
This analysis calculates the enrichment score, z-score, based on how often spots
of different categorical labels (specified with column_name
) lies
adjacent to each other. The observed number of edges between the labels is
then compared with the results from a set number of permutations (chosen
with n_permutations
), allowing the calculation of a z-score.
The z-score will indicate if a label pair is overrepresented or underrepresented
as compared to what would be expected to see by chance.
The output of this function is a tibble table that for each label pair contains
information about the observed number of edges (edges
), the mean of the permuted
results (perm_mean
), the standard deviation of the permuted results (perm_sd
),
and the z-score (z_score
).
See also
Other spatial-methods:
CorSpatialFeatures()
,
CutSpatialNetwork()
,
DisconnectRegions()
,
GetSpatialNetwork()
,
RadialDistance()
,
RegionNeighbors()
,
RunLabelAssortativityTest()
,
RunLocalG()
Examples
# \donttest{
library(semla)
# Read data
se <- readRDS(system.file("extdata/mousebrain",
"se_mbrain",
package = "semla"))
# Generate clusters
se <- se |>
NormalizeData() |>
ScaleData() |>
FindVariableFeatures() |>
RunPCA() |>
FindNeighbors(reduction = "pca", dims = 1:30) |>
FindClusters()
#> Centering and scaling data matrix
#> PC_ 1
#> Positive: Nrgn, Olfm1, Cck, Nptxr, Rtn1, Snca, Nov, Tmsb4x, Lamp5, Egr1
#> Crym, Cpne6, Coro1a, Arc, Hpca, Sst, Nr4a1, Npy, Chgb, Neurod6
#> Snap25, Myh7, Uchl1, Eef1a2, Cort, Grp, Stmn2, Rprm, Spink8, Mfge8
#> Negative: Mbp, Plp1, Apod, Mobp, Ptgds, Mog, Mal, Mag, Cnp, Aldh1a1
#> Opalin, Tcf7l2, Ddc, Lhx1os, Slc6a3, Ret, Th, Slc18a2, Sncg, Drd2
#> Chrna6, Slc10a4, Spp1, En1, Dlk1, Calb2, Hbb-bs, Pvalb, Col1a2, Tnnt1
#> PC_ 2
#> Positive: Myoc, Gfap, Col1a2, Fmod, Slc13a4, Hba-a1, Hbb-bt, Slc6a20a, Hba-a2, Hbb-bs
#> Acta2, Tagln, Mgp, Ogn, Vtn, H2-Aa, Lyz2, Cd74, Myl9, Dcn
#> Myh11, Ptgds, Cytl1, H2-Eb1, Hmgcs2, Clu, Mfge8, Emp1, Npy, Cnn1
#> Negative: Th, Uchl1, Slc18a2, En1, Slc10a4, Slc6a3, Chrna6, Stmn2, Ret, Snap25
#> Drd2, Dlk1, Ddc, Scg2, Sncg, Eef1a2, Rtn1, Chga, Pcp4, Calb2
#> Mobp, Mbp, Chgb, Fabp5, Plp1, Pvalb, Mog, Mal, Mag, Snca
#> PC_ 3
#> Positive: Trbc2, Arc, Egr1, Myl4, Nr4a1, Mbp, Mobp, Pvalb, Plp1, Opalin
#> Mog, Mal, Cnp, Mag, Snap25, Lamp5, Ighm, Tcf7l2, Cplx3, Tgm3
#> Ighg2c, Pcp4, Hpca, Ly6d, Eef1a2, Tnnt1, Chga, Neurod6, Prph, Ctgf
#> Negative: Nnat, Slc18a2, Dlk1, Slc6a3, Slc10a4, En1, Th, Chrna6, Dcn, Sncg
#> Cpne7, Drd2, Ddc, Ret, Hpcal1, Ecel1, Cpne6, Col1a2, Trh, Calb2
#> Cd24a, Fmod, Mgp, Snca, Lypd1, Slc13a4, Fibcd1, Crym, Spink8, Slc6a20a
#> PC_ 4
#> Positive: Nr4a1, Arc, Lamp5, Egr1, Myl4, Trbc2, Chrna6, Tagln, En1, Snap25
#> Th, Slc18a2, Acta2, Col1a2, Myh11, Fmod, Hba-a2, Slc10a4, Slc6a3, Slc13a4
#> Ret, Tgm3, Hbb-bt, Slc6a20a, Ighm, Hbb-bs, Hba-a1, Vtn, Mgp, Drd2
#> Negative: Spink8, Fibcd1, Tmsb4x, Nnat, Lefty1, Crym, Cpne7, Nos1, Dcn, Cpne6
#> Grp, Homer3, Htr3a, Tac1, Fabp5, C1ql2, Trh, Opalin, Mog, Plp1
#> Mag, Calb2, Ecel1, Gfap, Cnp, Tcf7l2, Lypd1, Vgll3, Hpcal1, Mal
#> PC_ 5
#> Positive: Pcp4, Tcf7l2, Snap25, Uchl1, Eef1a2, Chga, Stmn2, Lhx1os, Calb2, Scg2
#> Fabp5, Bok, Prkcd, Pvalb, Cartpt, Chgb, Tnnt1, Gpx3, Slc20a1, Rtn1
#> C1ql2, Spp1, Hpcal1, Ptgds, Pitx2, Lypd1, Aldh1a1, Ecel1, Vtn, Nme7
#> Negative: En1, Chrna6, Th, Slc18a2, Slc10a4, Ddc, Lefty1, Arc, Slc6a3, Neurod6
#> Grp, Nov, Lamp5, Fibcd1, Nr4a1, Drd2, Spink8, Vip, Myl4, Ret
#> Egr1, Dlk1, Nrgn, Gfap, Trbc2, Tgm3, Myh7, Tac2, Sncg, Npy
#> Computing nearest neighbor graph
#> Computing SNN
#> Modularity Optimizer version 1.3.0 by Ludo Waltman and Nees Jan van Eck
#>
#> Number of nodes: 2560
#> Number of edges: 107827
#>
#> Running Louvain algorithm...
#> Maximum modularity in 10 random starts: 0.8228
#> Number of communities: 12
#> Elapsed time: 0 seconds
# Run Neigborhood Enrichment Analysis
res <- RunNeighborhoodEnrichmentTest(object = se,
column_name = "seurat_clusters",
n_permutations = 100,
nCores = 1)
#>
#> ── Running Neighborhood Enrichment Analysis ──
#>
#> ℹ Generating neighborhood adjacency data from observed labels in column 'seurat_clusters'
#> ✔ Observed label adjacency calculations complete
#> ℹ Generating neighborhood adjacency data from randomized labels
#> ✔ Randomized label adjacency calculations complete from 100 iterations
#> ✔ Scores calculated for each label pair and returned as output tibble
res |> arrange(desc(abs(z_score)))
#> # A tibble: 132 × 7
#> label_1 label_2 label_label z_score edges perm_mean perm_sd
#> <fct> <fct> <chr> <dbl> <int> <dbl> <dbl>
#> 1 Label_0 Label_1 Label_0-Label_1 -22.0 1 403. 18.2
#> 2 Label_1 Label_0 Label_1-Label_0 -22.0 1 403. 18.2
#> 3 Label_0 Label_4 Label_0-Label_4 -20.6 4 292. 14.0
#> 4 Label_4 Label_0 Label_4-Label_0 -20.6 4 292. 14.0
#> 5 Label_0 Label_2 Label_0-Label_2 -20.0 2 367. 18.3
#> 6 Label_2 Label_0 Label_2-Label_0 -20.0 2 367. 18.3
#> 7 Label_0 Label_3 Label_0-Label_3 -17.4 13 344. 19.1
#> 8 Label_3 Label_0 Label_3-Label_0 -17.4 13 344. 19.1
#> 9 Label_1 Label_3 Label_1-Label_3 -16.5 41 294. 15.3
#> 10 Label_3 Label_1 Label_3-Label_1 -16.5 41 294. 15.3
#> # ℹ 122 more rows
# }