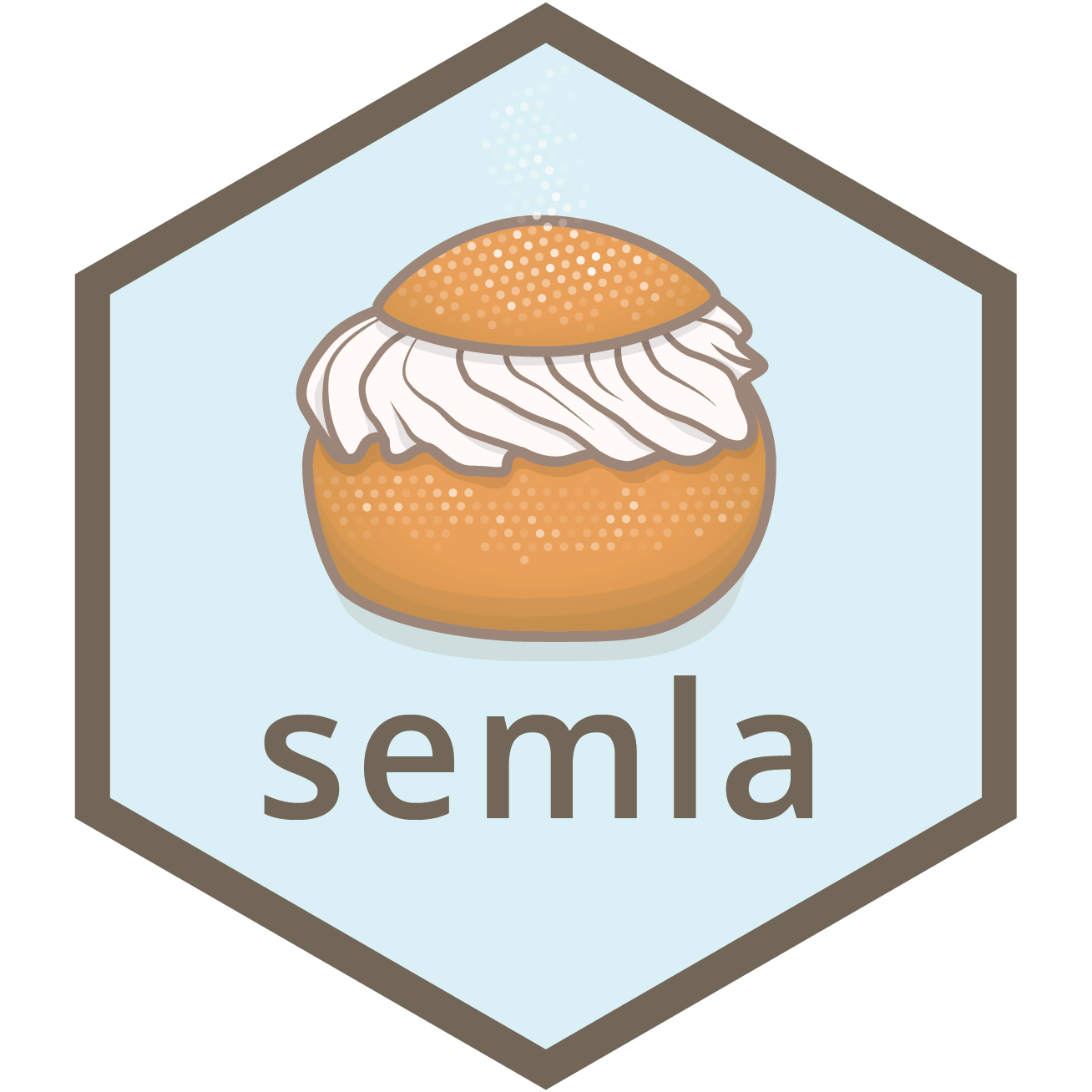
Create dataset
Last compiled: 19 March 2025
create_object.Rmd
In this notebook, we’ll have a look at how you can create a
Seurat
object compatible with semla
.
Load coordinates
The Staffli
object requires image and coordinate data
that can be retrieved from the spaceranger output files.
Below we’ll use the mouse brain and mouse colon example data:
he_imgs <- c(system.file("extdata/mousebrain",
"spatial/tissue_lowres_image.jpg",
package = "semla"),
system.file("extdata/mousecolon",
"spatial/tissue_lowres_image.jpg",
package = "semla"))
spotfiles <- c(system.file("extdata/mousebrain",
"spatial/tissue_positions_list.csv",
package = "semla"),
system.file("extdata/mousecolon",
"spatial/tissue_positions_list.csv",
package = "semla"))
jsonfiles <- c(system.file("extdata/mousebrain",
"spatial/scalefactors_json.json",
package = "semla"),
system.file("extdata/mousecolon",
"spatial/scalefactors_json.json",
package = "semla"))
When loading coordinates from multiple samples, you need to change
the barcode IDs so that the suffix matches their respective sampleID.
For instance, a barcode in sample 1 might be called CATACAAAGCCGAACC-1
and in sample 2 the same barcode should be CATACAAAGCCGAACC-2. The
coordinates
tibble below contains the barcode IDs, the
pixel coordinates and a column with the sampleIDs.
semla
provides LoadSpatialCoordinates
to
make the task a little easier. Note that the suffix for the barcodes
have changed for sample 2.
# Read coordinates
coordinates <- LoadSpatialCoordinates(spotfiles) |>
select(all_of(c("barcode", "pxl_col_in_fullres", "pxl_row_in_fullres", "sampleID")))
## ℹ Loading coordinates:
## → Finished loading coordinates for sample 1
## → Finished loading coordinates for sample 2
## ℹ Collected coordinates for 5164 spots.
head(coordinates, n = 2)
## # A tibble: 2 × 4
## barcode pxl_col_in_fullres pxl_row_in_fullres sampleID
## <chr> <int> <int> <int>
## 1 CATACAAAGCCGAACC-1 6086 4117 1
## 2 CTGAGCAAGTAACAAG-1 5062 4472 1
tail(coordinates, n = 2)
## # A tibble: 2 × 4
## barcode pxl_col_in_fullres pxl_row_in_fullres sampleID
## <chr> <int> <int> <int>
## 1 GAAGGAGTCGAGTGCG-2 4854 6829 2
## 2 TGACCAAATCTTAAAC-2 4957 6829 2
# Check number of spots per sample
table(coordinates$sampleID)
##
## 1 2
## 2560 2604
Fetch image info
Next, we’ll fetch meta data for the sample H&E images. Here, the
tibble must contain the width and height of he_imgs
as well
as a sampleID column. Use LoadImageInfo
to load the image
info:
# Create image_info
image_info <- LoadImageInfo(he_imgs)
image_info
## # A tibble: 2 × 9
## format width height colorspace matte filesize density sampleID type
## <chr> <int> <int> <chr> <lgl> <int> <chr> <chr> <chr>
## 1 JPEG 565 600 sRGB FALSE 106233 +72x+72 1 tissue_lowres
## 2 JPEG 600 541 sRGB FALSE 141710 +72x+72 2 tissue_lowres
Define image dimensions
The jsonfiles
contain scaling factors that allows us to
find the original H&E image dimensions. We can load the scale
factors with read_json
and store them in a tibble. The
tibble should also contain a ‘sampleID’ column. Use
LoadScaleFactors
to load the scaling factors:
# Read scalefactors
scalefactors <- LoadScaleFactors(jsonfiles)
scalefactors
## # A tibble: 2 × 5
## spot_diameter_fullres tissue_hires_scalef fiducial_diameter_fullres
## <dbl> <dbl> <dbl>
## 1 143. 0.104 215.
## 2 67.0 0.202 108.
## # ℹ 2 more variables: tissue_lowres_scalef <dbl>, sampleID <chr>
Once we have the scale factors, we can add additional columns to
image_info
which specify the dimensions of the original
H&E images. Use UpdateImageInfo
to update
image_info
with the scaling factors:
# Add additional columns to image_info using scale factors
image_info <- UpdateImageInfo(image_info, scalefactors)
image_info
## # A tibble: 2 × 10
## format width height full_width full_height colorspace filesize density
## <chr> <int> <int> <dbl> <dbl> <chr> <int> <chr>
## 1 JPEG 565 600 18120. 19242. sRGB 106233 +72x+72
## 2 JPEG 600 541 9901. 8927. sRGB 141710 +72x+72
## # ℹ 2 more variables: sampleID <chr>, type <chr>
Finally, we are ready to create the Staffli
object. Here
we’ll provide the he_imgs
so that we can load the images
later with LoadImages
. The coordinates
are
stored in the meta_data
slot. image_info
will
be used by the plot functions provided in semla
to define
the dimensions of the plot area.
# Create Staffli object
staffli_object <- CreateStaffliObject(imgs = he_imgs,
meta_data = coordinates,
image_info = image_info,
scalefactors = scalefactors)
staffli_object
## An object of class Staffli
## 5164 spots across 2 samples.
Use a Staffli
object in Seurat
Now let’s see how we can incorporate our Staffli
object
in Seurat
. First, we’ll load the gene expression matrices
for our samples and merge them.
# Get paths for expression matrices
expr_matrix_files <- he_imgs <- c(system.file("extdata/mousebrain",
"filtered_feature_bc_matrix.h5",
package = "semla"),
system.file("extdata/mousecolon",
"filtered_feature_bc_matrix.h5",
package = "semla"))
Before merging the matrices, it’s important to rename the barcodes so
that they match the barcodes in Staffli_object
.
exprMatList <- lapply(seq_along(expr_matrix_files), function(i) {
exprMat <- Seurat::Read10X_h5(expr_matrix_files[i])
colnames(exprMat) <- gsub(pattern = "-\\d*", # Replace barcode suffix with sampleID
replacement = paste0("-", i),
x = colnames(exprMat))
return(exprMat)
})
# Merge expression matrices
mergedExprMat <- SeuratObject::RowMergeSparseMatrices(exprMatList[[1]], exprMatList[[2]])
Alternatively, use the LoadAndMergeMatrices
function
from semla
:
mergedExprMat <- LoadAndMergeMatrices(samplefiles = expr_matrix_files, verbose = FALSE)
# Create Seurat object
se <- SeuratObject::CreateSeuratObject(counts = mergedExprMat)
Rearrange Staffli
object to match Seurat
object. If the barcode are mismatched, make sure to subset the two
object to contain intersecting barcodes.
staffli_object@meta_data <- staffli_object@meta_data[match(colnames(se), staffli_object@meta_data$barcode), ]
Check that the Staffli
object matches
Seurat
object:
## [1] TRUE
Place Staffli
object in the Seurat
object:
se@tools$Staffli <- staffli_object
Now the semla
functions should be compatible with the
Seurat
object!
MapFeatures(se, features = "nFeature_RNA")
se <- LoadImages(se, verbose = FALSE)
MapFeatures(se, features = "nFeature_RNA", image_use = "raw", override_plot_dims = TRUE)
Package version
-
semla
: 1.3.1
Session info
## R version 4.3.3 (2024-02-29)
## Platform: aarch64-apple-darwin20.0.0 (64-bit)
## Running under: macOS 15.3
##
## Matrix products: default
## BLAS/LAPACK: /Users/javierescudero/miniconda3/envs/r-semla/lib/libopenblas.0.dylib; LAPACK version 3.12.0
##
## locale:
## [1] en_US.UTF-8/en_US.UTF-8/en_US.UTF-8/C/en_US.UTF-8/en_US.UTF-8
##
## time zone: Europe/Stockholm
## tzcode source: internal
##
## attached base packages:
## [1] stats graphics grDevices utils datasets methods base
##
## other attached packages:
## [1] tibble_3.2.1 jsonlite_1.8.8 magick_2.8.3 semla_1.3.1
## [5] ggplot2_3.5.0 dplyr_1.1.4 SeuratObject_5.0.1 Seurat_4.3.0.1
##
## loaded via a namespace (and not attached):
## [1] RColorBrewer_1.1-3 rstudioapi_0.15.0 magrittr_2.0.3
## [4] spatstat.utils_3.0-5 farver_2.1.1 rmarkdown_2.26
## [7] fs_1.6.3 ragg_1.3.3 vctrs_0.6.5
## [10] ROCR_1.0-11 memoise_2.0.1 spatstat.explore_3.2-6
## [13] htmltools_0.5.7 forcats_1.0.0 sass_0.4.8
## [16] sctransform_0.4.1 parallelly_1.38.0 KernSmooth_2.23-22
## [19] bslib_0.6.1 htmlwidgets_1.6.4 desc_1.4.3
## [22] ica_1.0-3 plyr_1.8.9 plotly_4.10.4
## [25] zoo_1.8-12 cachem_1.0.8 igraph_2.0.2
## [28] mime_0.12 lifecycle_1.0.4 pkgconfig_2.0.3
## [31] Matrix_1.6-3 R6_2.5.1 fastmap_1.1.1
## [34] fitdistrplus_1.1-11 future_1.34.0 shiny_1.8.0
## [37] digest_0.6.34 colorspace_2.1-0 patchwork_1.2.0
## [40] tensor_1.5 irlba_2.3.5.1 textshaping_0.3.7
## [43] labeling_0.4.3 progressr_0.14.0 fansi_1.0.6
## [46] spatstat.sparse_3.0-3 httr_1.4.7 polyclip_1.10-6
## [49] abind_1.4-5 compiler_4.3.3 bit64_4.0.5
## [52] withr_3.0.0 highr_0.10 MASS_7.3-60
## [55] tools_4.3.3 lmtest_0.9-40 httpuv_1.6.14
## [58] future.apply_1.11.1 goftest_1.2-3 glue_1.7.0
## [61] dbscan_1.1-12 nlme_3.1-164 promises_1.2.1
## [64] grid_4.3.3 Rtsne_0.17 cluster_2.1.6
## [67] reshape2_1.4.4 generics_0.1.3 hdf5r_1.3.10
## [70] gtable_0.3.4 spatstat.data_3.0-4 tidyr_1.3.1
## [73] data.table_1.15.2 sp_2.1-3 utf8_1.2.4
## [76] spatstat.geom_3.2-9 RcppAnnoy_0.0.22 ggrepel_0.9.5
## [79] RANN_2.6.1 pillar_1.9.0 stringr_1.5.1
## [82] spam_2.10-0 later_1.3.2 splines_4.3.3
## [85] lattice_0.22-5 bit_4.0.5 survival_3.5-8
## [88] deldir_2.0-4 tidyselect_1.2.0 miniUI_0.1.1.1
## [91] pbapply_1.7-2 knitr_1.45 gridExtra_2.3
## [94] scattermore_1.2 xfun_0.42 matrixStats_1.2.0
## [97] stringi_1.8.3 lazyeval_0.2.2 yaml_2.3.8
## [100] evaluate_0.23 codetools_0.2-19 cli_3.6.2
## [103] uwot_0.1.16 xtable_1.8-4 reticulate_1.35.0
## [106] systemfonts_1.0.5 munsell_0.5.0 jquerylib_0.1.4
## [109] Rcpp_1.0.12 globals_0.16.3 spatstat.random_3.2-3
## [112] zeallot_0.1.0 png_0.1-8 parallel_4.3.3
## [115] ellipsis_0.3.2 pkgdown_2.0.7 dotCall64_1.1-1
## [118] listenv_0.9.1 viridisLite_0.4.2 scales_1.3.0
## [121] ggridges_0.5.6 leiden_0.4.3.1 purrr_1.0.2
## [124] rlang_1.1.3 cowplot_1.1.3 shinyjs_2.1.0